CICERO PERSPECTIVE
AI Isn’t the Strategy—It’s the Feedback Loop: Why Optimization Now Starts After Deployment
What to consider
Legacy organizations still treat AI like software. But in today’s AI-enabled ops, the real value comes not from implementation, but from iteration.
Too many companies still approach AI initiatives with a “build and deploy” mindset—treating models like static products. But unlike traditional systems, AI performance depends on what happens after launch. Optimization has become continuous, driven by feedback loops that must be operationalized just like finance or compliance workflows. For organizations to extract ROI from AI, the strategy must evolve from “implementation” to “adaptation.”
The Shift: From One-Time Launches to Continuous Optimization
The AI maturity curve is accelerating, but so is the frustration. A recent survey found that 70% of companies using AI see minimal or no financial impact. Why? Because most deploy a model, assume it’s “done,” and move on. But AI systems degrade over time without active feedback, retraining, and contextual adjustments. Unlike software, AI’s accuracy and utility are probabilistic—not deterministic.
This isn’t a theoretical problem. In supply chain, predictive AI models built on 2022 volatility are now outdated in 2025’s flatter demand environment. In fraud detection, adversaries evolve faster than static models. Optimization must be a service layer, not a project closeout.
The New Mandate: Operationalizing Feedback Loops
Optimization now requires four repeatable functions:
1. Signal Capture — Identify the right performance signals post-deployment (accuracy drift, cost per insight, latency bottlenecks).
2. Interpretation Layer — Use business context to map what signal deviations mean operationally.
3. Model Tuning/Replacement — Develop pipelines for quick iteration without full rebuilds.
4. Human-AI Collaboration — Build in loop closures from frontline staff who spot misfires AI can’t detect.
Case in Point: Contact Center Intelligence
One client—a large healthcare payer—deployed a generative AI assistant to support call reps. Initial gains were promising: a 23% reduction in average call times. But three months in, call satisfaction scores dipped. The cause? The AI over-summarized nuanced issues. Without feedback from reps, the system misprioritized brevity over clarity.
The team built an ongoing signal feedback loop, tying sentiment tracking, manual agent overrides, and post-call review into an optimization layer. The result: sustained gains, with call time reductions returning alongside improved CSAT.
Why This Matters Now
The explosion of AI usage—especially with LLMs—has outpaced most organizations’ ability to govern improvement. What separates top performers is not what they build, but how they evolve it. Optimization is no longer a tweak—it’s the product.
Cicero/MGT is helping clients reframe AI adoption as a living capability, not a finite deliverable. We partner with organizations to embed feedback-driven AI optimization into operations, governance, and P&L impact.
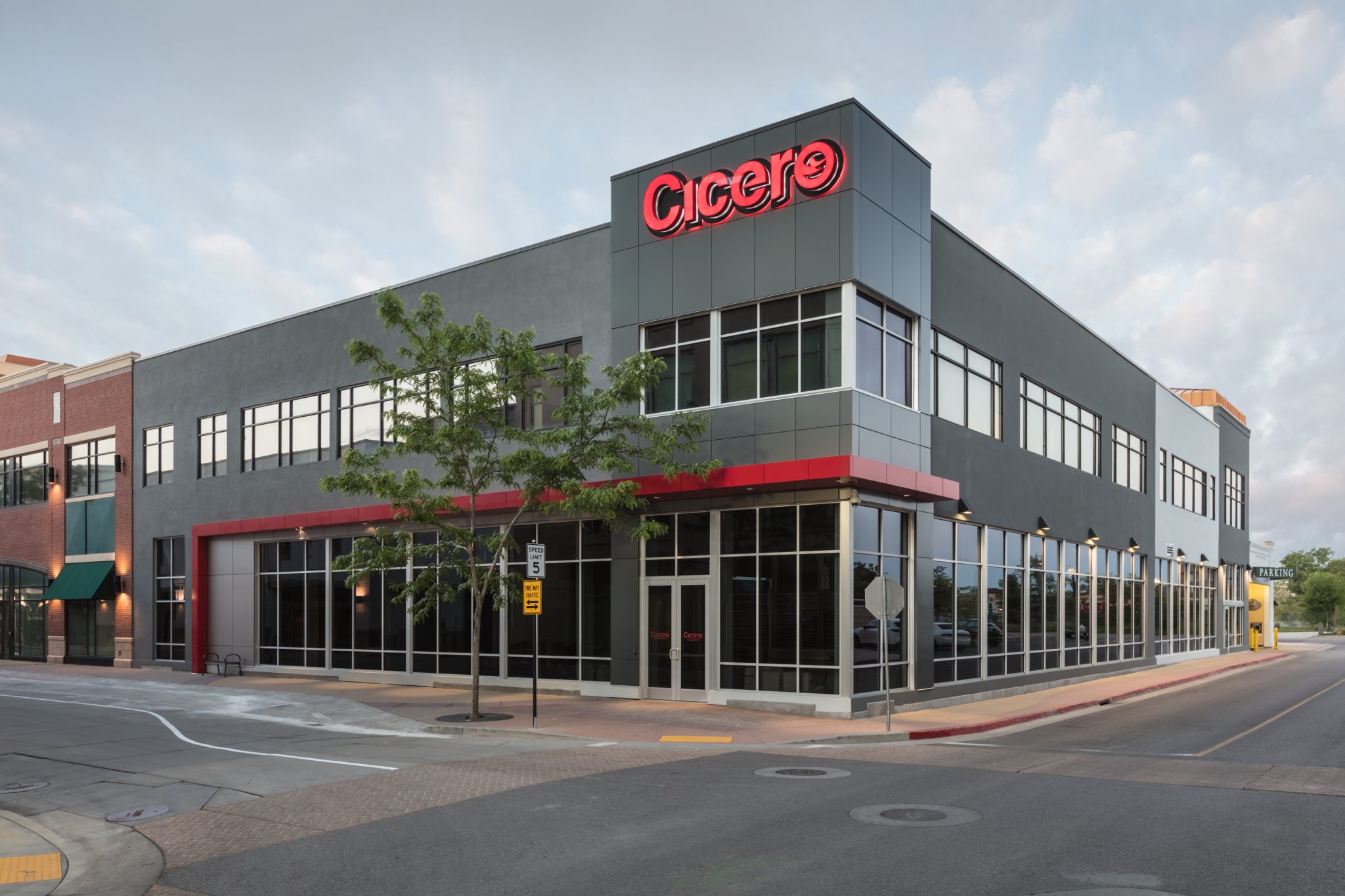
Start a Conversation
Thank you for your interest in Cicero Group. Please select from the options below to get in touch with us.